Alternating Direction Method of Multipliers for Machine Learning
Zhouchen Lin author Huan Li author Cong Fang author
Format:Paperback
Publisher:Springer Verlag, Singapore
Published:17th Jun '23
Currently unavailable, and unfortunately no date known when it will be back
This paperback is available in another edition too:
- Hardback£119.99(9789811698392)
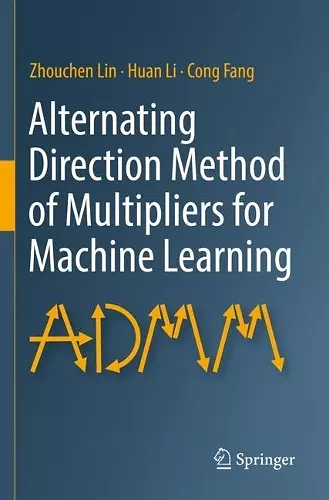
Machine learning heavily relies on optimization algorithms to solve its learning models. Constrained problems constitute a major type of optimization problem, and the alternating direction method of multipliers (ADMM) is a commonly used algorithm to solve constrained problems, especially linearly constrained ones. Written by experts in machine learning and optimization, this is the first book providing a state-of-the-art review on ADMM under various scenarios, including deterministic and convex optimization, nonconvex optimization, stochastic optimization, and distributed optimization. Offering a rich blend of ideas, theories and proofs, the book is up-to-date and self-contained. It is an excellent reference book for users who are seeking a relatively universal algorithm for constrained problems. Graduate students or researchers can read it to grasp the frontiers of ADMM in machine learning in a short period of time.
“This book is a valuable reference for researchers and graduate students in the field of optimization, statistics, machine learning and signal processing. It provides an excellent summary of the state of the art for theoretical research. … The book is strongly recommended as an auxiliary textbook for graduate students and researchers in multiple fields … . Each chapter of this volume provides a rich collection of related references, including a number of recent ones.” (Haydar Akca, zbMATH 1502.68010, 2023)
ISBN: 9789811698422
Dimensions: unknown
Weight: unknown
263 pages
2022 ed.