Federated Learning
Yang Liu author Qiang Yang author Yong Cheng author Yan Kang author Tianjian Chen author Han Yu author
Format:Paperback
Publisher:Springer International Publishing AG
Published:19th Dec '19
Currently unavailable, and unfortunately no date known when it will be back
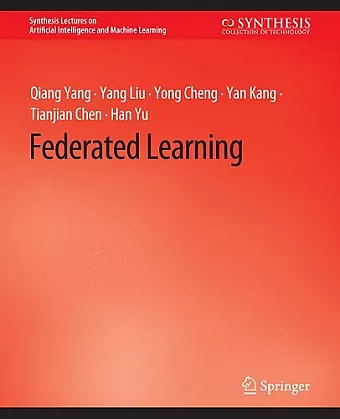
How is it possible to allow multiple data owners to collaboratively train and use a shared prediction model while keeping all the local training data private?
Traditional machine learning approaches need to combine all data at one location, typically a data center, which may very well violate the laws on user privacy and data confidentiality. Today, many parts of the world demand that technology companies treat user data carefully according to user-privacy laws. The European Union's General Data Protection Regulation (GDPR) is a prime example. In this book, we describe how federated machine learning addresses this problem with novel solutions combining distributed machine learning, cryptography and security, and incentive mechanism design based on economic principles and game theory. We explain different types of privacy-preserving machine learning solutions and their technological backgrounds, and highlight some representative practical use cases. We show how federated learning can become the foundation of next-generation machine learning that caters to technological and societal needs for responsible AI development and application.
ISBN: 9783031004575
Dimensions: unknown
Weight: unknown
189 pages