Deep Learning in Multi-step Prediction of Chaotic Dynamics
From Deterministic Models to Real-World Systems
Giorgio Guariso author Fabio Dercole author Matteo Sangiorgio author
Format:Paperback
Publisher:Springer Nature Switzerland AG
Published:15th Feb '22
Currently unavailable, and unfortunately no date known when it will be back
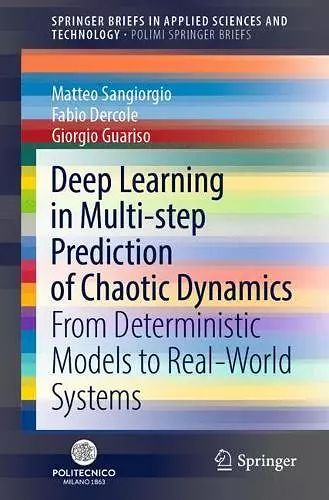
The book represents the first attempt to systematically deal with the use of deep neural networks to forecast chaotic time series. Differently from most of the current literature, it implements a multi-step approach, i.e., the forecast of an entire interval of future values. This is relevant for many applications, such as model predictive control, that requires predicting the values for the whole receding horizon. Going progressively from deterministic models with different degrees of complexity and chaoticity to noisy systems and then to real-world cases, the book compares the performances of various neural network architectures (feed-forward and recurrent). It also introduces an innovative and powerful approach for training recurrent structures specific for sequence-to-sequence tasks. The book also presents one of the first attempts in the context of environmental time series forecasting of applying transfer-learning techniques such as domain adaptation.
ISBN: 9783030944810
Dimensions: unknown
Weight: unknown
104 pages
1st ed. 2021