Multi-faceted Deep Learning
Models and Data
Akka Zemmari editor Jenny Benois-Pineau editor
Format:Hardback
Publisher:Springer Nature Switzerland AG
Published:20th Oct '21
Currently unavailable, and unfortunately no date known when it will be back
This hardback is available in another edition too:
- Paperback£149.99(9783030744809)
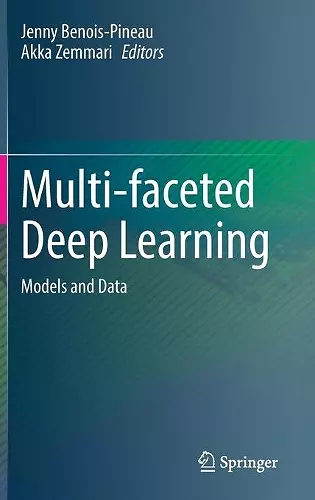
This book covers a large set of methods in the field of Artificial Intelligence - Deep Learning applied to real-world problems. The fundamentals of the Deep Learning approach and different types of Deep Neural Networks (DNNs) are first summarized in this book, which offers a comprehensive preamble for further problem–oriented chapters.
The most interesting and open problems of machine learning in the framework of Deep Learning are discussed in this book and solutions are proposed. This book illustrates how to implement the zero-shot learning with Deep Neural Network Classifiers, which require a large amount of training data. The lack of annotated training data naturally pushes the researchers to implement low supervision algorithms. Metric learning is a long-term research but in the framework of Deep Learning approaches, it gets freshness and originality. Fine-grained classification with a low inter-class variability is a difficult problem for any classification tasks. This book presents how it is solved, by using different modalities and attention mechanisms in 3D convolutional networks.
Researchers focused on Machine Learning, Deep learning, Multimedia and Computer Vision will want to buy this book. Advanced level students studying computer science within these topic areas will also find this book useful.
ISBN: 9783030744779
Dimensions: unknown
Weight: 658g
316 pages
1st ed. 2021