Missing Data in Longitudinal Studies
Strategies for Bayesian Modeling and Sensitivity Analysis
Michael J Daniels author Joseph W Hogan author
Format:Hardback
Publisher:Taylor & Francis Inc
Published:11th Mar '08
Currently unavailable, and unfortunately no date known when it will be back
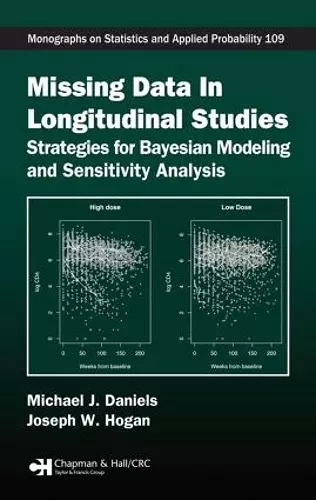
Drawing from the authors’ own work and from the most recent developments in the field, Missing Data in Longitudinal Studies: Strategies for Bayesian Modeling and Sensitivity Analysis describes a comprehensive Bayesian approach for drawing inference from incomplete data in longitudinal studies. To illustrate these methods, the authors employ several data sets throughout that cover a range of study designs, variable types, and missing data issues.
The book first reviews modern approaches to formulate and interpret regression models for longitudinal data. It then discusses key ideas in Bayesian inference, including specifying prior distributions, computing posterior distribution, and assessing model fit. The book carefully describes the assumptions needed to make inferences about a full-data distribution from incompletely observed data. For settings with ignorable dropout, it emphasizes the importance of covariance models for inference about the mean while for nonignorable dropout, the book studies a variety of models in detail. It concludes with three case studies that highlight important features of the Bayesian approach for handling nonignorable missingness.
With suggestions for further reading at the end of most chapters as well as many applications to the health sciences, this resource offers a unified Bayesian approach to handle missing data in longitudinal studies.
The authors combine their expertise in longitudinal data and Bayesian inference to missing data problems to give an overview of methods that can be used in various longitudinal studies. … the examples … are very helpful to illustrate the potential of the theory.
—Michael Bücker, Statistical Papers (2011) 52
Daniels and Hogan’s is the first to explicitly focus on missing data in the context of longitudinal studies. … I found the book extremely clear and illuminating. It is well written, with comprehensive and up-to-date references. The use of example datasets from a number of epidemiological and clinical studies illustrates how the methods and strategies being advocated can be applied in real-life settings. … an extremely valuable resource both to applied statisticians who are faced with analyzing longitudinal data subject to missingness and methodological researchers in the area.
—Jonathan Bartlett, Statistics in Medicine, 2011, 30
… They [the authors] have gone further than anyone else in developing methods for the not missing at random (NMAR) case. … The focus on longitudinal studies will attract many readers. … this book is an excellent introduction and is also a first-rate treatment of cutting-edge topics. …
—Paul D. Allison, University of Pennsylvania, Significance, September 2010
This text is the only Bayesian textbook that provides a contemporary and comprehensive treatment of Bayesian approaches to a common and critically important topic. The authors provide a scholarly treatment of Bayesian inference and supplement their treatise with concrete practical examples. The writing is clear, precise and interesting. A particularly innovative and enormously useful contribution is the authors’ formalization of sensitivity analyses. They distinguish between local and global sensitivity analyses, providing the reader with examples of each. I have used the techniques proposed in the text with much success, teaching people the importance of separating what is observed from what is assumed. I strongly endorse this book.
—Sharon-Lise Normand, Harvard School of Public Health, Boston, Massachusetts, USA
…the book under review appears to be the first reference that solely focuses on Bayesian approaches to handle missing data in longitudinal studies. … Overall I think this is a well-written technical monograph. The preliminary sections on longitudinal data analysis, Bayesian statistics, and missing data … are well written and serve to make this book a self-contained reference. The models presented to analyze missing data in longitudinal studies cover many ideas from the current literature, and some of the methods are at the cutting edge of research. The book will probably have greatest appeal to statisticians with a research interest in missing data. Although I also think applied biostatisticians who like to use Bayesian approaches and in particular WinBUGS will find this book very useful.
—Journal of Biopharmaceutical Statistics, 2009
…a timely and thorough review of this maturing research area. … The book is comprehensive in covering models for both continuous and discrete outcomes from both the pattern mixture and selection modeling perspectives. … The book’s composition offers much to admire. The writing is clear and direct, the notation is sensible and consistent, and tables and figures are simple and uncluttered. Typos are mercifully rare … Biostatisticians who seek a clear and thorough overview of the state of knowledge in this area would do well to make this excellent book their first stop.
—Biometrics, March 2009
ISBN: 9781584886099
Dimensions: unknown
Weight: 640g
324 pages