Model-Based Machine Learning
Format:Hardback
Publisher:Taylor & Francis Inc
Published:26th Oct '23
Currently unavailable, and unfortunately no date known when it will be back
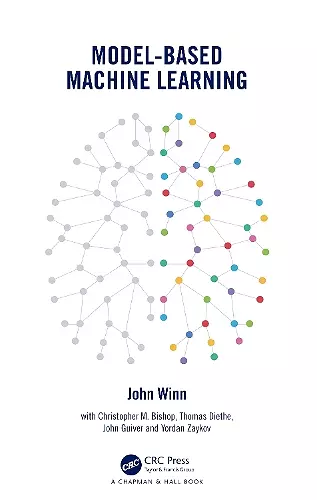
Today, machine learning is being applied to a growing variety of problems in a bewildering variety of domains. A fundamental challenge when using machine learning is connecting the abstract mathematics of a machine learning technique to a concrete, real world problem. This book tackles this challenge through model-based machine learning which focuses on understanding the assumptions encoded in a machine learning system and their corresponding impact on the behaviour of the system.
The key ideas of model-based machine learning are introduced through a series of case studies involving real-world applications. Case studies play a central role because it is only in the context of applications that it makes sense to discuss modelling assumptions. Each chapter introduces one case study and works through step-by-step to solve it using a model-based approach. The aim is not just to explain machine learning methods, but also showcase how to create, debug, and evolve them to solve a problem.
Features:
- Explores the assumptions being made by machine learning systems and the effect these assumptions have when the system is applied to concrete problems.
- Explains machine learning concepts as they arise in real-world case studies.
- Shows how to diagnose, understand and address problems with machine learning systems.
- Full source code available, allowing models and results to be reproduced and explored.
- Includes optional deep-dive sections with more mathematical details on inference algorithms for the interested reader. <
"I read Model-based Machine Learning with enthusiasm and curiosity, finding it well writtenand captivating. This book helps understand some of the most common models and it can beused by both beginners and more experienced individuals. I particularly appreciated that itpushes readers to think critically about the assumptions, sometimes hidden, their validity, andtheir consequences on the results obtained. As people’s lives are influenced by machine-madedecisions, understanding the assumptions that affect the behavior of a a machine learningalgorithm and making sure that it is transparent, interpretable and fair is an essential skill tohave for anyone who is responsible for any stage of a model’s development."
-Emanuela Furfaro, in Journal of the American Statistical Association, October 2024
ISBN: 9781498756815
Dimensions: unknown
Weight: 960g
455 pages