Predicting Hotspots
Using Machine Learning to Understand Civil Conflict
John David author Atin Basuchoudhary author James T Bang author Tinni Sen author
Format:Hardback
Publisher:Lexington Books
Published:15th Aug '18
Currently unavailable, and unfortunately no date known when it will be back
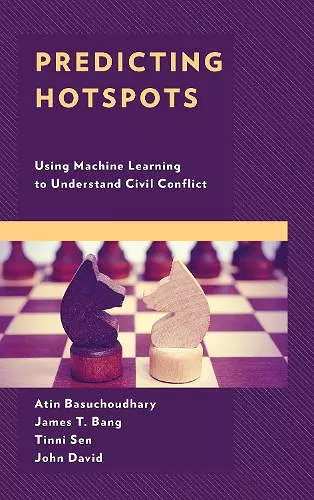
This book should be useful to anyone interested in identifying the causes of civil conflict and doing something to end it. It even suggests a pathway for the lay reader. Civil conflict is a persistent source of misery to humankind. Its study, however, lacks a comprehensive theory of its causes. Nevertheless, the question of cooperation or conflict is at the heart of political economy. This book introduces Machine Learning to explore whether there even is a unified theory of conflict, and if there is, whether it is a ‘good’ one. A good theory is one that not only identifies the causes of conflict, but also identifies those causes that predict conflict. Machine learning algorithms use out of sample techniques to choose between competing hypotheses about the sources of conflict according to their predictive accuracy. This theoretically agnostic ‘picking’ has the added benefit of offering some protection against many of the problems noted in the current literature; the tangled causality between conflict and its correlates, the relative rarity of civil conflict at a global level, missing data, and spectacular statistical assumptions. This book argues that the search for a unified theory of conflict must begin among these more predictive sources of civil conflict. In fact, in the book, there is a clear sense that game theoretic rational choice models of bargaining/commitment failure predict conflict better than any other approach. In addition, the algorithms highlight the fact that conflict is path dependent - it tends to continue once started. This is intuitive in many ways but is roundly ignored as a matter of science. It should not. Further, those causes of conflict that best predict conflict can be used as policy levers to end or prevent conflict. This book should therefore be of interest to military and civil leaders engaged in ending civil conflict. Last, though not least, the book highlights how the sources of conflict affect conflict. This additional insight may allow the crafting of policies that match a country’s specific circumstance.
In Predicting Hotspots: Using Machine Learning to Understand Civil Conflict James T. Bang, Atin Basuchoudhary, John David, and Tinni Sen provide a vital contribution to the social scientific study of civil wars and other forms of violence within states. Whereas most theoretical and empirical studies of intrastate conflicts emphasize the correlates or the causes of violence, this book offers a variety of standard and innovative methodologies to best predict future civil wars. The book is a must-have for scholars and policymakers concerned about predicting future civil wars and what can be done to prevent them. -- Charles H. Anderton, College of the Holy Cross
Predicting Hotspots: Using Machine Learning to Understand Civil Conflict is an ambitious and successful demonstration of how machine learning can be employed towards a holistic understanding of civil conflict. It provides a concise and intuitive introduction to machine learning using conflict data. In so doing, the top socioeconomic predictors of civil conflict are identified. Of equal or greater value is the authors’ insightful discussion of how their findings can better inform policy making and theoretical model selection. -- Dann Arce, University of Texas at Dallas
Basuchoudhary, Tinni, Bang and David make a compelling case for using machine learning to predict conflict. The book is a timely and very welcome addition to our knowledge on the correlates of conflict. -- Günther Schulze, Professor of Economics, University of Freiburg
ISBN: 9781498520676
Dimensions: 231mm x 159mm x 20mm
Weight: 454g
178 pages