Ensemble Methods
Foundations and Algorithms
Format:Hardback
Publisher:Taylor & Francis Inc
Published:6th Jun '12
Currently unavailable, and unfortunately no date known when it will be back
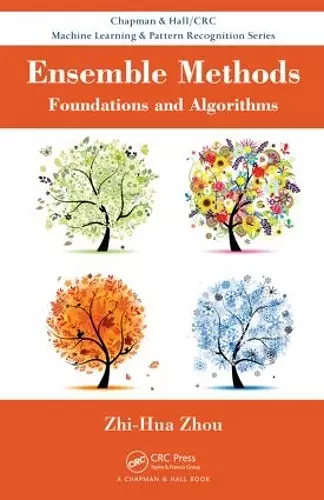
An up-to-date, self-contained introduction to a state-of-the-art machine learning approach, Ensemble Methods: Foundations and Algorithms shows how these accurate methods are used in real-world tasks. It gives you the necessary groundwork to carry out further research in this evolving field.
After presenting background and terminology, the book covers the main algorithms and theories, including Boosting, Bagging, Random Forest, averaging and voting schemes, the Stacking method, mixture of experts, and diversity measures. It also discusses multiclass extension, noise tolerance, error-ambiguity and bias-variance decompositions, and recent progress in information theoretic diversity.
Moving on to more advanced topics, the author explains how to achieve better performance through ensemble pruning and how to generate better clustering results by combining multiple clusterings. In addition, he describes developments of ensemble methods in semi-supervised learning, active learning, cost-sensitive learning, class-imbalance learning, and comprehensibility enhancement.
"… a valuable contribution to theoretical and practical ensemble learning. The material is very well presented, preliminaries and basic knowledge are discussed in detail, and many illustrations and pseudo-code tables help to understand the facts of this interesting field of research. Therefore, the book will become a helpful tool for practitioners working in the field of machine learning or pattern recognition as well as for students of engineering or computer sciences at the graduate and postgraduate level. I heartily recommend this book!"
—IEEE Computational Intelligence Magazine, February 2013
"While the book is rather written for a machine learning and pattern recognition audience, the terminology is well explained and therefore also easily understandable for readers from other areas. In general the book is well structured and written and presents nicely the different ideas and approaches for combining single learners as well as their strengths and limitations."
—Klaus Nordhausen, International Statistical Review (2013), 81
"Professor Zhou’s book is a comprehensive introduction to ensemble methods in machine learning. It reviews the latest research in this exciting area. I learned a lot reading it!"
—Thomas G. Dietterich, Professor and Director of Intelligent Systems Research, Oregon State University, Corvallis, USA; ACM Fellow; and Founding President of the International Machine Learning Society
"This is a timely book. Right time and right book … with an authoritative but inclusive style that will allow many readers to gain knowledge on the topic."
—Fabio Roli, University of Cagliari, Italy
ISBN: 9781439830031
Dimensions: unknown
Weight: 521g
236 pages