A Computational Approach to Statistical Learning
Michael Kane author Taylor Arnold author Bryan W Lewis author
Format:Hardback
Publisher:Taylor & Francis Ltd
Published:29th Jan '19
Currently unavailable, and unfortunately no date known when it will be back
This hardback is available in another edition too:
- Paperback£45.99(9780367570613)
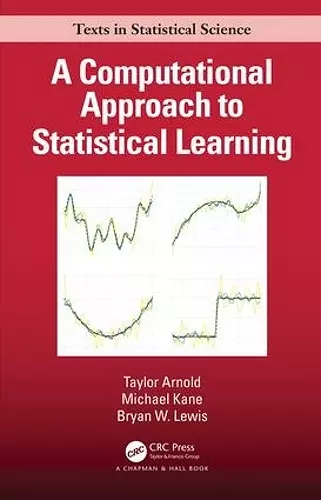
A Computational Approach to Statistical Learning gives a novel introduction to predictive modeling by focusing on the algorithmic and numeric motivations behind popular statistical methods. The text contains annotated code to over 80 original reference functions. These functions provide minimal working implementations of common statistical learning algorithms. Every chapter concludes with a fully worked out application that illustrates predictive modeling tasks using a real-world dataset.
The text begins with a detailed analysis of linear models and ordinary least squares. Subsequent chapters explore extensions such as ridge regression, generalized linear models, and additive models. The second half focuses on the use of general-purpose algorithms for convex optimization and their application to tasks in statistical learning. Models covered include the elastic net, dense neural networks, convolutional neural networks (CNNs), and spectral clustering. A unifying theme throughout the text is the use of optimization theory in the description of predictive models, with a particular focus on the singular value decomposition (SVD). Through this theme, the computational approach motivates and clarifies the relationships between various predictive models.
"As best as I can determine, ‘A Computational Approach to Statistical Learning’ (CASL) is unique among R books devoted to statistical learning and data science. Other popular texts…cover much of the same ground, and include extensive R code implementing statistical models. What makes CASL different is the unifying mathematical structure underlying the presentation and the focus on the computations themselves…CASL’s great strengths are the use linear algebra to provide a coherent, unifying mathematical framework for explaining a wide class of models, a lucid writing style that appeals to geometric intuition, clear explanations of many details that are mostly glossed over in more superficial treatments, the inclusion of historical references, and R code that is tightly integrated into the text. The R code is extensive, concise without being opaque, and in many cases, elegant. The code illustrates R’s advantages for developing statistical algorithms as well as its power to present versatile and compelling visualizations…CASL ought to appeal to anyone working in data science or machine learning seeking a sophisticated understanding of both the theoretical basis and efficient algorithms underlying a modern approach to computational statistics."
~Joe Rickert, RStudio
"The ‘literate programming’ style is my favorite part of this book (borrowing the term from Don Knuth). It would be well suited for an engineer seeking to understand the implementations and ideas behind these statistical models. Real code beats pseudocode, because one can easily tweak and experiment with it…The other part I especially like is the development of neural nets based on extending the models previously introduced in the text. This takes some of the mystery out of neural nets and makes them more accessible to a statistician studying them for the first time... I would happily buy this book for my own reference and self-study... I’m not aware of any books that are written at this level that combines the motivation, the mathematics and the code in such a nice way. If I ever happen to be teaching a course on this material, then I would definitely teach from this book."
~Clark Fitzgerald, University of California, Davis
"I think the book is quite clearly written and covers really important things to consider that can help optimize model building. The book does a really great job of following its theme throughout and explicitly mentioning why they are explaining something the way they explain it. Reading the book, it is clear they considered how all the parts the included (at least the chapters I read) fit into the broader scope of the book's goal."
~Justin Post, North Carolina State University
"As best as I can determine, ‘A Computational Approach to Statistical Learning’ (CASL) is unique among R books devoted to statistical learning and data science. Other popular texts…cover much of the same ground, and include extensive R code implementing statistical models. What makes CASL different is the unifying mathematical structure underlying the presentation and the focus on the computations themselves…CASL’s great strengths are the use linear algebra to provide a coherent, unifying mathematical framework for explaining a wide class of models, a lucid writing style that appeals to geometric intuition, clear explanations of many details that are mostly glossed over in more superficial treatments, the inclusion of historical references, and R code that is tightly integrated into the text. The R code is extensive, concise without being opaque, and in many cases, elegant. The code illustrates R’s advantages for developing statistical algorithms as well as its power to present versatile and compelling visualizations…CASL ought to appeal to anyone working in data science or machine learning seeking a sophisticated understanding of both the theoretical basis and efficient algorithms underlying a modern approach to computational statistics."
~Joe Rickert, RStudio
"The ‘literate programming’ style is my favorite part of this book (borrowing the term from Don Knuth). It would be well suited for an engineer seeking to understand the implementations and ideas behind these statistical models. Real code beats pseudocode, because one can easily tweak and experiment with it…The other part I especially like is the development of neural nets based on extending the models previously introduced in the text. This takes some of the mystery out of neural nets and makes them more accessible to a statistician studying them for the first time... I would happily buy this book for my own reference and self-study... I’m not aware of any books that are written at this level that combines the motivation, the mathematics and the code in such a nice way. If I ever happen to be teaching a course on this material, then I would definitely teach from this book."
~Clark Fitzgerald, University of California, Davis
"I think the book is quite clearly written and covers really important things to consider that can help optimize model building. The book does a really great job of following its theme throughout and explicitly mentioning why they are explaining something the way they explain it. Reading the book, it is clear they considered how all the parts the included (at least the chapters I read) fit into the broader scope of the book's goal."
~Justin Post, North Carolina State University
ISBN: 9781138046375
Dimensions: unknown
Weight: 683g
376 pages