Model-Based Processing
An Applied Subspace Identification Approach
Format:Hardback
Publisher:John Wiley & Sons Inc
Published:14th Jun '19
Currently unavailable, and unfortunately no date known when it will be back
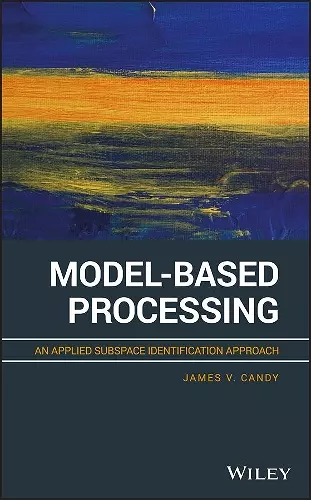
This book introduces subspace identification techniques for model-based signal processing, providing essential methods for engineers and students alike.
This book serves as a crucial link between the application of subspace-based methods for parameter estimation in signal processing and their use in subspace-based system identification within control systems. Model-Based Processing offers readers expert insights into the development of models designed for model-based signal processors (MBSP) using subspace identification techniques. The goal is to achieve effective model-based identification (MBID) while allowing readers to evaluate overall performance through validation and statistical analysis methods. The focus on subspace approaches to system identification problems equips readers with the skills needed to quickly identify models and apply them to a variety of processing challenges, including state estimation, tracking, detection, classification, controls, and communications.
The extraction of models from data is essential for numerous applications, from detecting submarines to pinpointing earthquake epicenters and controlling autonomous vehicles. These tasks require a solid understanding of the underlying processes and measurement instrumentation. Model-Based Processing emphasizes real-world solutions to diverse model development issues, showcasing how model-based subspace identification facilitates the extraction of models from measured data sequences, ranging from simple time series polynomials to complex parametric adaptive systems. The book also covers important topics such as Kalman filtering for various system types and practical processor designs, while linking model development to practical applications in model-based signal processing.
This resource is particularly beneficial for advanced undergraduate and graduate students in engineering and science, as well as engineers in both industry and academia. With numerous appendices, problem sets, case studies, and examples, Model-Based Processing provides a comprehensive guide to bridging the gap between statistical signal processing and subspace identification, making it an essential addition to any technical library.
ISBN: 9781119457763
Dimensions: 234mm x 158mm x 31mm
Weight: 998g
544 pages