Mathematical Foundations of Infinite-Dimensional Statistical Models
Evarist Giné author Richard Nickl author
Format:Paperback
Publisher:Cambridge University Press
Published:25th Mar '21
Should be back in stock very soon
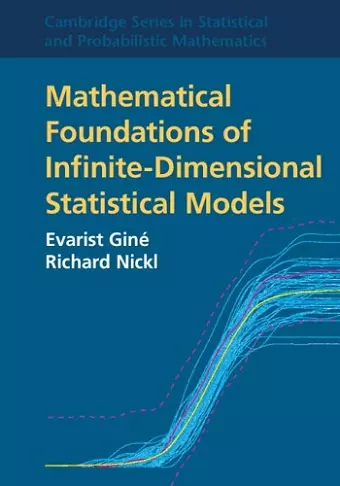
Now in paperback: the new classic on the theory of statistical inference in statistical models with an infinite-dimensional parameter space.
High-dimensional and nonparametric statistical models are ubiquitous in modern data science. This book develops a mathematically coherent and objective approach to statistical inference in such models, with a focus on function estimation problems arising from random samples or from Gaussian regression/signal in white noise problems.In nonparametric and high-dimensional statistical models, the classical Gauss–Fisher–Le Cam theory of the optimality of maximum likelihood estimators and Bayesian posterior inference does not apply, and new foundations and ideas have been developed in the past several decades. This book gives a coherent account of the statistical theory in infinite-dimensional parameter spaces. The mathematical foundations include self-contained 'mini-courses' on the theory of Gaussian and empirical processes, approximation and wavelet theory, and the basic theory of function spaces. The theory of statistical inference in such models - hypothesis testing, estimation and confidence sets - is presented within the minimax paradigm of decision theory. This includes the basic theory of convolution kernel and projection estimation, but also Bayesian nonparametrics and nonparametric maximum likelihood estimation. In a final chapter the theory of adaptive inference in nonparametric models is developed, including Lepski's method, wavelet thresholding, and adaptive inference for self-similar functions. Winner of the 2017 PROSE Award for Mathematics.
ISBN: 9781108994132
Dimensions: 251mm x 176mm x 36mm
Weight: 1270g
704 pages
Revised edition