Variational Bayesian Learning Theory
Masashi Sugiyama author Shinichi Nakajima author Kazuho Watanabe author
Format:Paperback
Publisher:Cambridge University Press
Published:6th Feb '25
Currently unavailable, and unfortunately no date known when it will be back
This paperback is available in another edition too:
- Hardback£123.00(9781107076150)
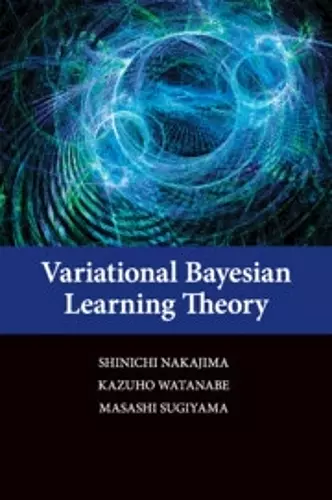
This introduction to the theory of variational Bayesian learning summarizes recent developments and suggests practical applications.
Designed for researchers and graduate students in machine learning, this book introduces the theory of variational Bayesian learning, a popular machine learning method, and suggests how to make use of it in practice. Detailed derivations allow readers to follow along without prior knowledge of the specific mathematical techniques.Variational Bayesian learning is one of the most popular methods in machine learning. Designed for researchers and graduate students in machine learning, this book summarizes recent developments in the non-asymptotic and asymptotic theory of variational Bayesian learning and suggests how this theory can be applied in practice. The authors begin by developing a basic framework with a focus on conjugacy, which enables the reader to derive tractable algorithms. Next, it summarizes non-asymptotic theory, which, although limited in application to bilinear models, precisely describes the behavior of the variational Bayesian solution and reveals its sparsity inducing mechanism. Finally, the text summarizes asymptotic theory, which reveals phase transition phenomena depending on the prior setting, thus providing suggestions on how to set hyperparameters for particular purposes. Detailed derivations allow readers to follow along without prior knowledge of the mathematical techniques specific to Bayesian learning.
'This book presents a very thorough and useful explanation of classical (pre deep learning) mean field variational Bayes. It covers basic algorithms, detailed derivations for various models (eg matrix factorization, GLMs, GMMs, HMMs), and advanced theory, including results on sparsity of the VB estimator, and asymptotic properties (generalization bounds).' Kevin Murphy, Research scientist, Google Brain
'This book is an excellent and comprehensive reference on the topic of Variational Bayes (VB) inference, which is heavily used in probabilistic machine learning. It covers VB theory and algorithms, and gives a detailed exploration of these methods for matrix factorization and extensions. It will be an essential guide for those using and developing VB methods.' Chris Williams, University of Edinburgh
ISBN: 9781107430761
Dimensions: unknown
Weight: unknown
559 pages