Federated Deep Learning for Healthcare
A Practical Guide with Challenges and Opportunities
Amandeep Kaur editor Chetna Kaushal editor Md Mehedi Hassan editor Si Thu Aung editor
Format:Hardback
Publisher:Taylor & Francis Ltd
Published:2nd Oct '24
Currently unavailable, and unfortunately no date known when it will be back
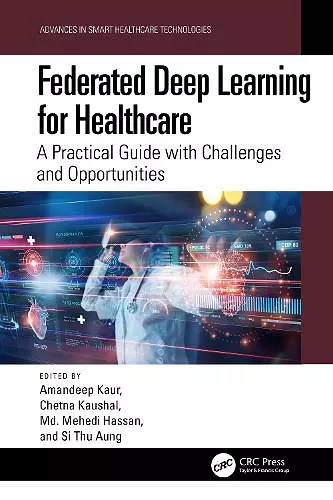
This book provides a practical guide to federated deep learning for healthcare including fundamental concepts, framework, and the applications comprising domain adaptation, model distillation, and transfer learning. It covers concerns in model fairness, data bias, regulatory compliance, and ethical dilemmas. It investigates several privacy-preserving methods such as homomorphic encryption, secure multi-party computation, and differential privacy. It will enable readers to build and implement federated learning systems that safeguard private medical information.
Features:
- Offers a thorough introduction of federated deep learning methods designed exclusively for medical applications.
- Investigates privacy-preserving methods with emphasis on data security and privacy.
- Discusses healthcare scaling and resource efficiency considerations.
- Examines methods for sharing information among various healthcare organizations while retaining model performance.
This book is aimed at graduate students and researchers in federated learning, data science, AI/machine learning, and healthcare.
ISBN: 9781032689555
Dimensions: unknown
Weight: 453g
252 pages