Dependence Models via Hierarchical Structures
Format:Hardback
Publisher:Cambridge University Press
Published:27th Mar '25
Currently unavailable, currently targeted to be due back around 31st May 2025, but could change
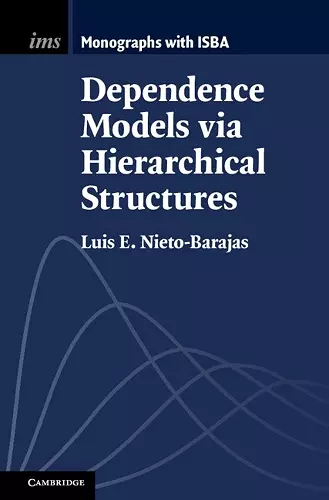
Learn to construct your own dependence models with this step-by-step look at models in a Bayesian analysis context.
Intended for senior undergraduate and postgraduate students, this text explores how to construct dependence models including exchangeable, Markov, temporal and spatial models. Readers are empowered to be creative and construct their own dependence models. Examples appear throughout, and multiple applications with data and code are provided.Bringing together years of research into one useful resource, this text empowers the reader to creatively construct their own dependence models. Intended for senior undergraduate and postgraduate students, it takes a step-by-step look at the construction of specific dependence models, including exchangeable, Markov, moving average and, in general, spatio-temporal models. All constructions maintain a desired property of pre-specifying the marginal distribution and keeping it invariant. They do not separate the dependence from the marginals and the mechanisms followed to induce dependence are so general that they can be applied to a very large class of parametric distributions. All the constructions are based on appropriate definitions of three building blocks: prior distribution, likelihood function and posterior distribution, in a Bayesian analysis context. All results are illustrated with examples and graphical representations. Applications with data and code are interspersed throughout the book, covering fields including insurance and epidemiology.
'Luis Nieto-Barajas has been making wonderful contributions to the Bayesian nonparametrics literature for many years, and it is exciting to see this new book on the important topic of inducing dependence in statistical models via intricate hierarchical structures. I plan to use this book as a reference for my students.' David B. Dunson, Duke University
'The book introduces Bayesian statistical modeling and inference from an unusual, but very meaningful perspective. The underlying organizing principle is based on increasingly more complex levels of modelling dependence subject to pre-specified marginals. The discussion starts with i.i.d. sampling and conjugate prior/likelihood pairs, continues with exchangeable models, moving on with Markov dependence, and finally arbitrary levels of dependence, including temporal and spatial dependence as special cases. The book could serve as a wonderful text for a second look at Bayesian statistical inference, from an unusual perspective. Using dependence structure as the main paradigm allows another view of familiar inference approaches. It is a great and inspiring text.' Peter Müller, University of Texas at Austin
'This book presents a step-by-step approach to constructing dependence models with pre-specified marginals, covering a wide range of discrete-time stochastic processes, along with practical applications. It is a highly valuable and enriching resource for graduate students and researchers alike.' Igor Prünster, University of Bocconi
'This monograph will be of interest to graduate or advanced undergraduate students that are looking for principled and elegant ways to construct models with stochastic dependence. The text goes from the simplest scenario (exchangeability) to more complicated spatio-temporal models. Many references and concrete examples are introduced and discussed in detail.' Fernando A. Quintana, Pontificia Universidad Católica de Chile
ISBN: 9781009584111
Dimensions: unknown
Weight: 362g
149 pages