Large-Scale Inverse Problems and Quantification of Uncertainty
David Keyes editor Luis Tenorio editor Matthias Heinkenschloss editor George Biros editor Omar Ghattas editor Bani Mallick editor Bart van Bloemen Waanders editor Lorenz Biegler editor
Format:Hardback
Publisher:John Wiley & Sons Inc
Published:5th Nov '10
Currently unavailable, and unfortunately no date known when it will be back
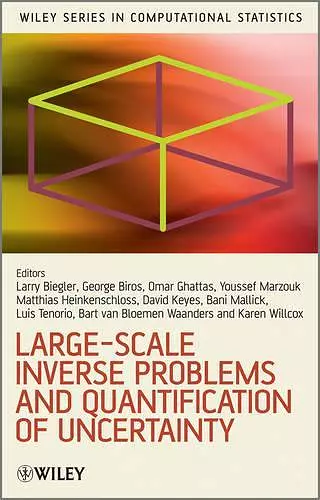
This book focuses on computational methods for large-scale statistical inverse problems and provides an introduction to statistical Bayesian and frequentist methodologies. Recent research advances for approximation methods are discussed, along with Kalman filtering methods and optimization-based approaches to solving inverse problems. The aim is to cross-fertilize the perspectives of researchers in the areas of data assimilation, statistics, large-scale optimization, applied and computational mathematics, high performance computing, and cutting-edge applications.
The solution to large-scale inverse problems critically depends on methods to reduce computational cost. Recent research approaches tackle this challenge in a variety of different ways. Many of the computational frameworks highlighted in this book build upon state-of-the-art methods for simulation of the forward problem, such as, fast Partial Differential Equation (PDE) solvers, reduced-order models and emulators of the forward problem, stochastic spectral approximations, and ensemble-based approximations, as well as exploiting the machinery for large-scale deterministic optimization through adjoint and other sensitivity analysis methods.
Key Features:
- Brings together the perspectives of researchers in areas of inverse problems and data assimilation.
- Assesses the current state-of-the-art and identify needs and opportunities for future research.
- Focuses on the computational methods used to analyze and simulate inverse problems.
- Written by leading experts of inverse problems and uncertainty quantification.
Graduate students and researchers working in statistics, mathematics and engineering will benefit from this book.
ISBN: 9780470697436
Dimensions: 236mm x 158mm x 23mm
Weight: 748g
400 pages