Dimensionality Reduction in Machine Learning
Snehashish Chakraverty editor Jamal Amani Rad editor Kourosh Parand editor
Format:Paperback
Publisher:Elsevier Science & Technology
Published:5th Feb '25
£145.99
Supplier delay - available to order, but may take longer than usual.
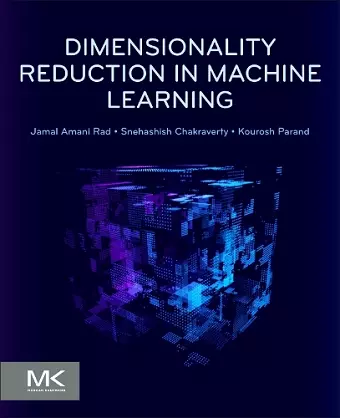
Dimensionality Reduction in Machine Learning covers both the mathematical and programming sides of dimension reduction algorithms, comparing them in various aspects. Part One provides an introduction to Machine Learning and the Data Life Cycle, with chapters covering the basic concepts of Machine Learning, essential mathematics for Machine Learning, and the methods and concepts of Feature Selection. Part Two covers Linear Methods for Dimension Reduction, with chapters on Principal Component Analysis and Linear Discriminant Analysis. Part Three covers Non-Linear Methods for Dimension Reduction, with chapters on Linear Local Embedding, Multi-dimensional Scaling, and t-distributed Stochastic Neighbor Embedding. Finally, Part Four covers Deep Learning Methods for Dimension Reduction, with chapters on Feature Extraction and Deep Learning, Autoencoders, and Dimensionality reduction in deep learning through group actions. With this stepwise structure and the applied code examples, readers become able to apply dimension reduction algorithms to different types of data, including tabular, text, and image data.
ISBN: 9780443328183
Dimensions: unknown
Weight: 450g
330 pages