Conformal Prediction for Reliable Machine Learning
Theory, Adaptations and Applications
Vladimir Vovk editor Vineeth Balasubramanian editor Shen-Shyang Ho editor
Format:Paperback
Publisher:Elsevier Science & Technology
Published:10th Jun '14
Currently unavailable, and unfortunately no date known when it will be back
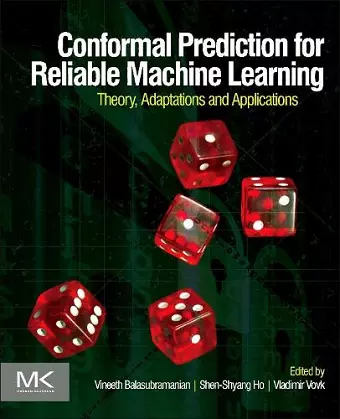
An introduction to the theory of the conformal prediction framework, with practical applications in medicine, finance, and more
The conformal predictions framework is a recent development in machine learning that can associate a reliable measure of confidence with a prediction in any real-world pattern recognition application, including risk-sensitive applications such as medical diagnosis, face recognition, and financial risk prediction. Conformal Predictions for Reliable Machine Learning: Theory, Adaptations and Applications captures the basic theory of the framework, demonstrates how to apply it to real-world problems, and presents several adaptations, including active learning, change detection, and anomaly detection. As practitioners and researchers around the world apply and adapt the framework, this edited volume brings together these bodies of work, providing a springboard for further research as well as a handbook for application in real-world problems.
"...captures the basic theory of the framework, demonstrates how to apply it to real-world problems, and presents several adaptations, including active learning, change detection, and anomaly detection." --Zentralblatt MATH, Sep-14 "...the book is highly recommended for people looking for formal machine learning techniques that can guarantee theoretical soundness and reliability." --Computing Reviews,December 4,2014 "This book captures the basic theory of the framework, demonstrates how the framework can be applied to real-world problems, and also presents several adaptations of the framework…" --HPCMagazine.com, August 2014
ISBN: 9780123985378
Dimensions: unknown
Weight: 700g
334 pages